Introducing New Audio Intelligence Models for Sentiment, Intent, and Topic Detection

Josh Fox
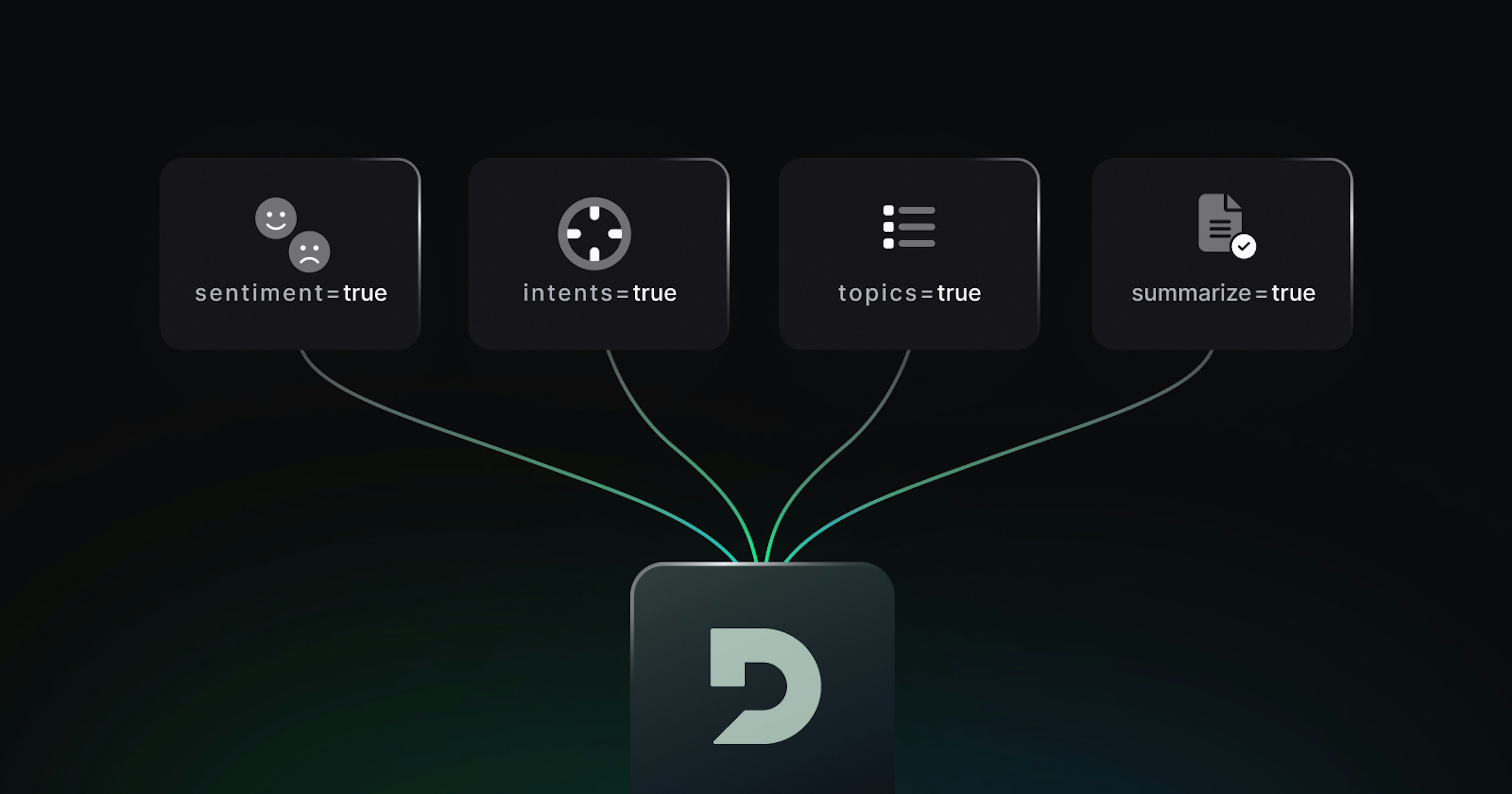
TL;DR:
We’ve enhanced our Voice AI platform with new audio intelligence models that provide spoken language understanding and speech analytics of conversational interactions
Our models are faster and more efficient than LLM-based alternatives and fine-tuned using more than 60K domain-specific conversations, allowing you to accurately score sentiment, recognize caller intent, and perform topic detection at scale.
Learn more about our new sentiment analysis, intent recognition, and topic detection models on our API Documentation, product page, and upcoming product showcase webinar.
Deepgram Audio Intelligence: Extracting insights and understanding from speech
We’re excited to announce a major expansion of our Audio Intelligence lineup since the launch of our first-ever task-specific language model (TSLM) for speech summarization last summer. This release includes models for high-level natural language understanding tasks for sentiment analysis, intent recognition, and topic detection.
In contrast to the hundred billion-parameter, general-purpose large language models (LLMs) from the likes of OpenAI and Google, our models are lightweight, purpose-driven, and fine-tuned on domain and task-specific conversational data sets. The result? Superior accuracy on specialized topics, lightning-fast speed, and low inference costs that make high throughput, low latency use cases viable such as contact center and sales enablement applications.
The Deepgram Voice AI Platform
In spite of continued investment in digital transformation and adoption of omnichannel customer service strategies, voice remains the most preferred and most heavily used customer communication channel. As a result, leading service organizations are increasingly turning to AI technologies like next-gen speech-to-text, advanced speech analytics, and conversational AI agents (i.e. voicebots) to maximize the efficiency and effectiveness of this important channel.
But transcribing voice interactions with fast, efficient, accurate speech recognition is only just the beginning. Enterprises need to know more than just who said what and when; they need rich insights about what was said and why. Deriving these insights in real-time and at scale is critical to improve agent handling and optimizing the next steps in any given interaction.
As a full stack voice AI platform provider, Deepgram is committed to developing the essential building blocks our customers need to create powerful voice AI experiences across a range of use cases. There are three main components of such a platform that correspond with the primary phases in a conversational interaction. These phases include:
Perceive: Using perceptive AI models like speech-to-text to accurately transcribe conversational audio into text.
Understand: Using abstractive AI models that implement intelligent natural and spoken language understanding tasks like summarization and sentiment analysis.
Interact: Using forms of generative AI models like text-to-speech and large language models (LLMs) to interact with human speakers just as they would with another person.