The ARC Benchmark: Evaluating LLMs' Reasoning Abilities
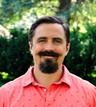
Brad Nikkel
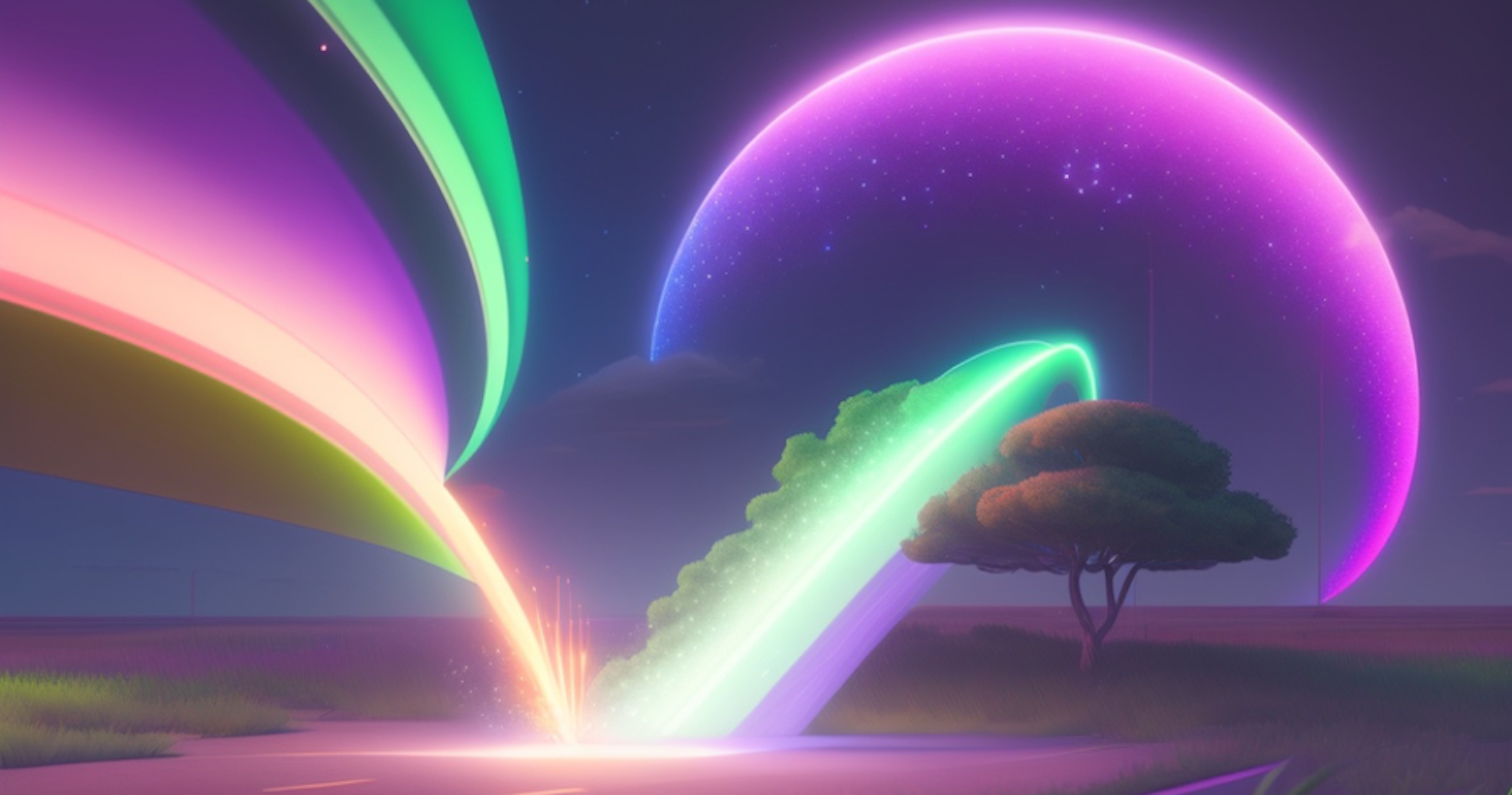
New large language models (LLMs) spring up on a near-weekly basis, often with accompanying bold claims about their abilities. To test these claims’ veracity, researchers carefully craft evaluation tasks (benchmarks) designed to challenge current state-of-the-art (SOTA) LLMs.
Goodhart’s law, often summed up as “When a measure becomes a target, it ceases to be a good measure," is widely applicable across domains but particularly salient toward LLMs, since machines and algorithms are now adept at recognizing and memorizing patterns at massive scale. Given Goodhart’s law, we ought to take LLM benchmarks with a grain of salt, but they’re still useful.
Because language is very multifaceted, different LLM benchmarks zero in on different language aspects, including how well LLMs answer questions, summarize text, retrieve information, analyze sentiment, and model language (among many other LLM capabilities). Since no single benchmark evaluates every aspect of language, testing LLMs on multiple benchmarks is common practice. This also prevents incentivizing LLMs to target a single benchmark, rendering that benchmark useless (by Goodhart’s law).
Hugging Face, an open-source championing artificial intelligence (AI) company, hosts a handy "Open LLM Leaderboard" that does just this, automatically evaluating open LLMs submitted to their Hub on several foundational benchmarks, measuring various reasoning and knowledge tasks in zero to 25-shot settings. Hugging Face’s four choice benchmarks are:
Over a four-part series, we’ll dig into each of these benchmarks to get a sense of what exactly Hugging Face’s Open LLM Leaderboard aims to evaluate and learn about what goes into designing challenging LLM benchmarks. First up, we’ll tackle the AI2 Reasoning Challenge (ARC).
Benchmarking Question-Answering Prowess: ARC
Perhaps the bare minimum we ask of our LLMs is accurate, informative answers (to our near-endless questions if that’s not asking too much). Current LLMs accomplish this pretty well, but it wasn’t always so.
In 2018, Clark et al. conceived the AI2 Reasoning Challenge to be a more demanding “knowledge and reasoning” test than similar question-answer (QA) benchmarks (at that time), like the Stanford Question Answering Dataset (SQuAD) and Stanford Natural Language Inference Dataset (SNLI).
With ARC, Clark et al. sought to push the field beyond existing, relatively easy QA benchmarks—often focused on merely retrieving factoids from passages—toward benchmarks that measure more important QA capacities like the reasoning, commonsense knowledge, and deep comprehension skill needed to answer difficult, complex questions. Making headway in the former entails better pattern matching while making headway in the latter entails engineering LLMs with more human-like reading comprehension—a more useful capability for many applications.
To pull this off, Clark et al. generated more complex questions than previous datasets. Their ARC dataset contains 7787 non-diagram, 4-way multiple-choice science questions designed for 3rd through 9th grade-level standardized tests. These questions—derived from numerous sources and targeting various knowledge types (e.g., spatial, experimental, algebraic, process, factual, structural, definition, and purpose)—are split into an “Easy Set” and a “Challenge Set.”
The Challenge Set contains 2590 questions that at least one of a retrieval-based and a word co-occurrence algorithm—the Information Retrieval (IR) Solver and the Pointwise Mutual Information (PMI) Solver—incorrectly answered. Because IR and PMI rely heavily on words’ co-occurrences, Clark et al. assumed that the questions IR and PMI faltered on were tough enough to merit more advanced QA models, earning them their Challenge Set designation.
Below is an example Easy Set and an example Challenge Set question to give you a sense of their difference: