LLMs, NLP, and NLU: What your words say about your mental health

Victoria Hseih
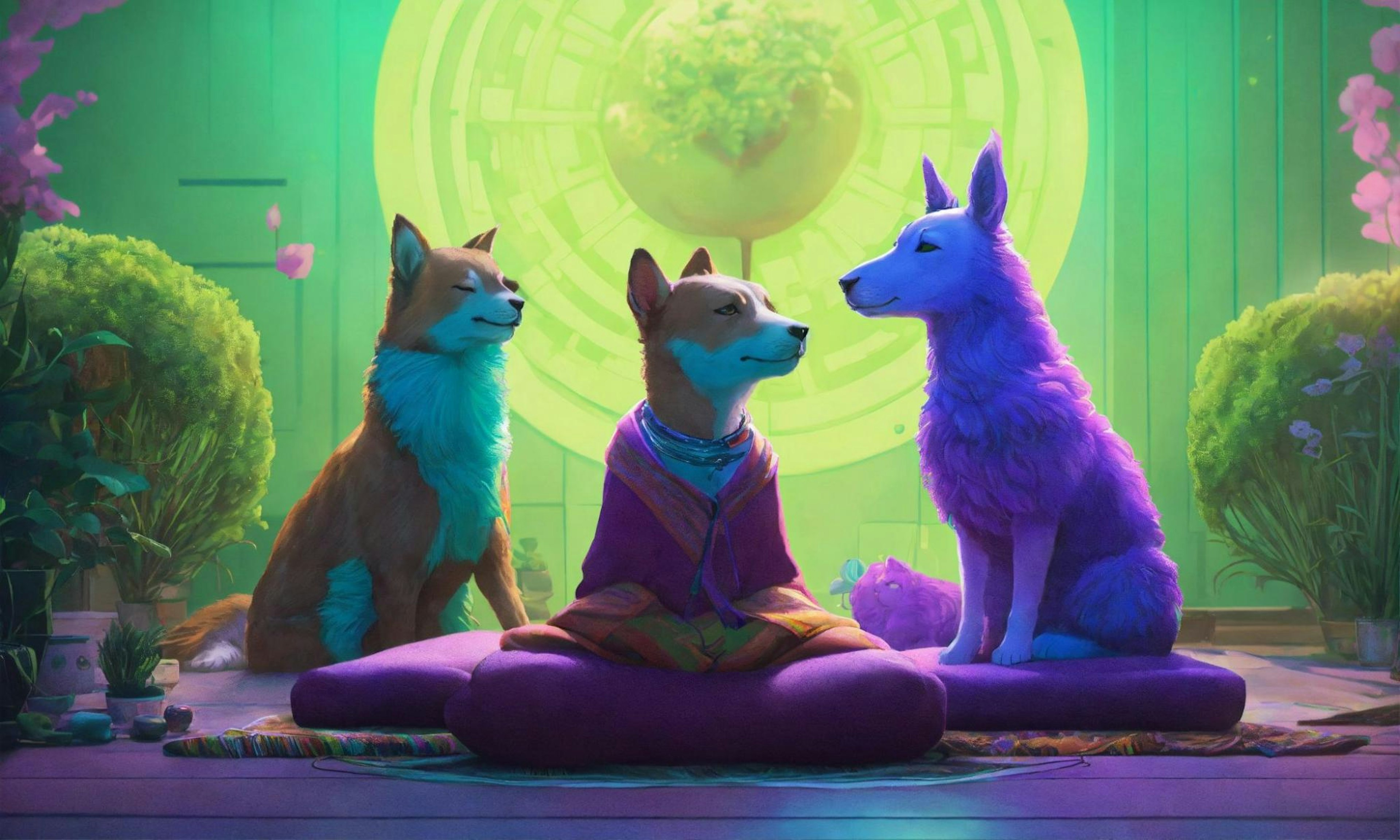
Researchers collected essays from depressed and depression-vulnerable college students and found differences in their work. Based on text analysis of self-submitted essays, the study found that students who are prone to depression tend to use “I” (e.g. “I am”, “I feel”, etc.) more frequently than their counterparts. One participant with depression often expressed negative emotions and then proceeded to backpedal or apologize for their negative emotions. Given the linguistic differences in these essays, is it possible to utilize linguistic analysis in mental health diagnostics?
In healthcare, natural language processing (NLP) has long been used to analyze electronic medical records to determine crucial events like drug-drug interactions. This type of textual analysis requires extensive annotation by experts and also a large number of definitions of concepts; however, when it comes to diagnosing mental health, there is now an increased focus on what is directly said by the patient as opposed to words filtered through physician notes.
Old School NLP in Mental Health: The Case of Schizophrenia
People experiencing psychosis (and related conditions) are likely to exhibit disordered use of language. As such, researchers in this corner of the mental health field have been using NLP to gain a better understanding of what people with these conditions are experiencing.
Schizophrenia is an example of this. Most of language, such as pronunciation, grammatical rules, and semantics, remains intact in people with schizophrenia but maintaining flow and cohesion across sentences and clauses can be impaired.
In the 1980s, Yale professor of psychiatry Ralph E. Hoffman and his colleagues created computational models of the language of people with schizophrenia, finding larger differences with the language of those with schizophrenia compared to those with other psychotic disorders. For example, in schizophrenia, basic discourse structure, or how a body of text is organized, is disturbed, while in mania, a person may switch from different discourse structures to another. Hoffman and his colleagues built an artificial neural network to simulate the breakdown of speech in schizophrenia in 2011 from this early research.
The first application of NLP, conducted by Brita Eldevag and her colleagues in 2007, to patient narratives was applying latent semantic analysis, an NLP technique examining relationships between the document and specific terms within it, to speech produced by patients undergoing treatment for schizophrenia. This technique differentiated speech in schizophrenia from the norm with 82% accuracy and from unaffected adult siblings with 86% accuracy.
Another technique used to determine coherence of speech in schizophrenia is linguistic graph theory, which maps out connections between words as edges. A machine learning classifier based on this was able to predict a schizophrenia diagnosis six months later with 92% accuracy after a first psychotic episode.
Thus, in the case of schizophrenia, scientists are able to model potential speech symptoms in schizophrenia and differentiate speech patterns from other psychotic disorders. But how does this apply to diagnostic tools for patients?
Use of NLP in Mental Health Diagnostic Tools
Researchers Zhang et al., in a 2022 paper published in Nature NPJ, conducted a survey of contemporary mental health screening methods, and found that 59% of mental illness detection methods utilize traditional machine learning, following the pipeline of data pre-processing to optimization and evaluation. The most frequently used features in this model are based on linguistic patterns that can be easily derived via text processing. These features include part-of-speech tagging, representing text as a bag-of-words, word count, and lengths of sentences and passages. For example, the Coh-Metrix tool assessed referential cohesion in at-risk youths for psychosis by analyzing written descriptions in response to visual prompts. It applied part-of-speech tagging to these narratives and identified root words and their morphological forms, like plurals, to determine relations across the text. It was found that patients with a clinical high risk for psychosis had less referential cohesion across sentences.
Another example is the use of Linguistic Inquiry and Word Count, a software for analyzing word use. Those with depression were found to use more words related to sadness compared to those with anxiety or even anxiety and depression through this software.
Furthermore, some automated assessments focus more on the acoustic features of language as opposed to the text itself. The use of acoustic measures that include speech intensity and duration are often altered in those with depression. For example, we see a lowered pitch and a slower speech rate in those with more severe depression. These features have the potential to be used in classification models to support the diagnosis of depressed individuals.
More specifically, other assessments use spectral features, which represents the association between the change in vocal tract shape and vocal movement. Mel Frequency Cepstral Coefficients, or MFCCs, are one example of a spectral feature; they collectively make up a short representation of a sound’s power spectrum. MFCCs can be used to classify the speech of individuals undergoing stress and distinguish individuals with depression.
Researchers are also pursuing the use of NLP approaches to support mental health diagnoses in non-clinical settings. Amongst 399 reviewed papers on machine and deep learning methods in mental health detection, 81% of these papers utilized social media as their source. For example, De Choudhury and his colleagues developed a classifier to estimate the risk of depression and utilized this to analyze interactions between Twitter users. It was found that depressed Twitter users were more likely to tweet late at night and respond less to other users’ tweets. Because of clear distinctions in behavior on social media between individuals without depression and individuals with depression, these NLP techniques could potentially support more rigorous therapy-based intervention.
NLP in Non-Clinical Settings
NLP may be implemented into non-clinical settings—like mobile applications and in-home smart speakers—to collect more granular data of a person’s speech and (at least somewhat) accurately predict if an individual is at risk for depression. This is useful for elderly patients who are unable to visit doctor’s offices and this system could passively monitor patients with mild cognitive impairments. For example, when elderly patients interact with Amazon Alexa or Google Home, their speech could be tracked to determine if they are at risk for mild cognitive impairment or depression. The speech could be measured in between doctor visits without interfering with the patient’s life.
Evidently, there are ethical concerns in the use of NLP for mental health diagnosis. There is a danger of clinical abuse as it is questionable how ethical it is to use models to entirely diagnose someone with a mental health disorder like psychosis. Furthermore, with the recent use of studies with extensive amounts of data from social media, it could be considered a violation of confidentiality. Users online may not have full and informed consent on using this data for future early diagnostic tools. Machine learning and NLP tools should not replace the patient-clinician relationship, rather they should enhance it.